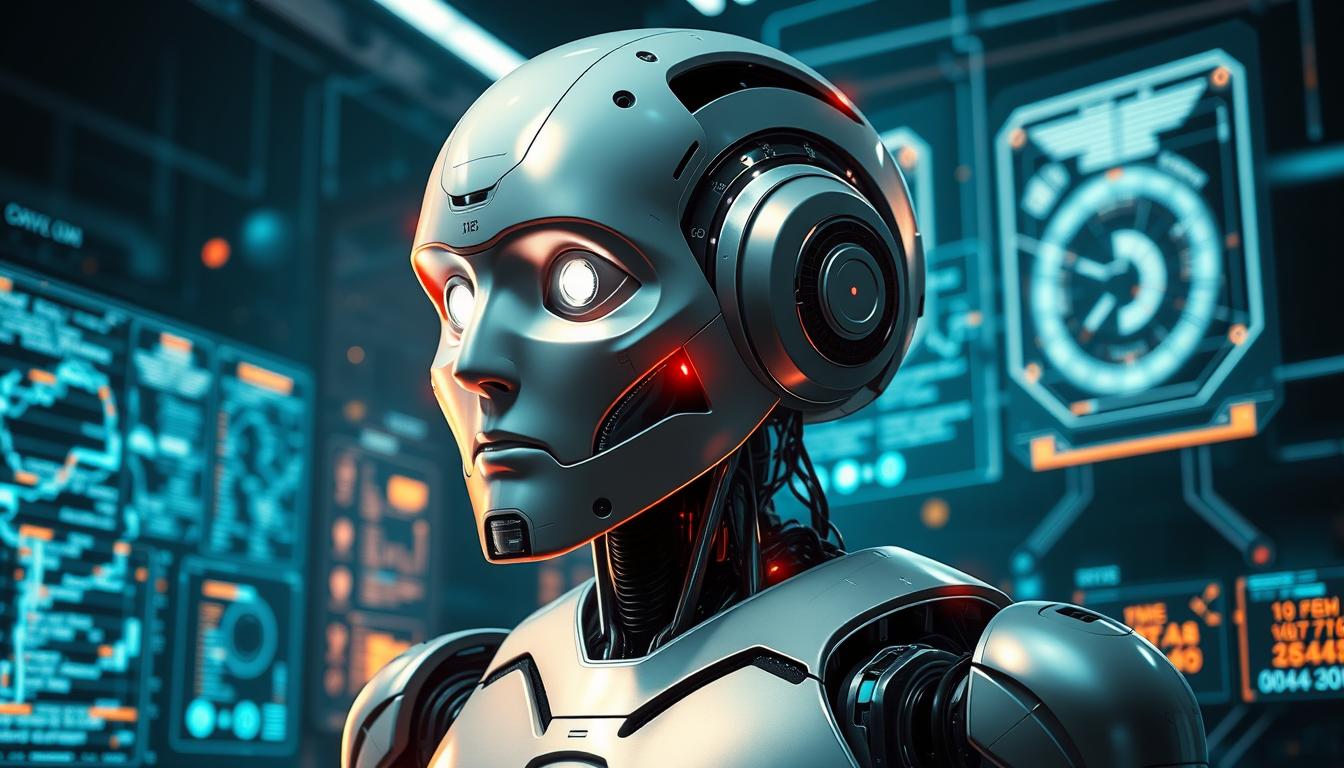
In early 2011, Watson beat Ken Jennings in Jeopardy. Watson scored $35,734 to Jennings’ $4,800 in the first game. This showed the power of artificial intelligence and cognitive computing.
Watson was trained on over 200 million pages of documents. This included dictionaries, encyclopedias, novels, plays, and the Bible. It was made to be a revolutionary system.
As you learn about Watson, you’ll see its impact on AI and cognitive computing. It has shaped the development of new technologies.
The Birth of IBM’s Watson: A Revolutionary Vision
IBM started researching AI in the 1950s. They made big strides, like beating chess grandmaster Garry Kasparov with Deep Blue. This led to the creation of IBM’s Watson, designed to play Jeopardy! using natural language processing and machine learning.
The Watson project was led by a team at IBM. They wanted to make a system that could answer complex questions. They used data analytics to handle lots of data and give precise answers. Their goal was to make a system that could get better over time, thanks to machine learning.
Watson’s development was a big step towards cognitive computing. This technology lets systems think like humans, giving more accurate answers. Watson’s use of natural language processing and machine learning helped it understand and analyze vast amounts of data, revealing new insights.
Origins of the Watson Project
The Watson project took four years to complete, from 2007 to 2011. During this time, IBM’s team worked hard to make a system that could play Jeopardy! and answer tough questions.
Initial Goals and Aspirations
The Watson project’s first aim was to compete in Jeopardy! and answer complex questions well. The team hoped to create a system that could learn and get better, using machine learning to improve its skills.
The Promise of Cognitive Computing
Watson’s development marked a big move towards cognitive computing. This technology lets systems think like humans, giving more accurate and detailed answers. Watson’s use of natural language processing and machine learning helped it understand and analyze vast amounts of data, revealing new insights.
Year | Event | Description |
---|---|---|
2007 | Watson project began | Development of Watson started, with a goal to compete in Jeopardy! |
2011 | Watson competed in Jeopardy! | Watson defeated Brad Rutter and Ken Jennings, the two highest-ranked players, in the Jeopardy! Challenge |
2013 | IBM Watson Developer Cloud launched | The IBM Watson Developer Cloud allowed for the availability of Watson technology as a development platform |
Triumph on Jeopardy: The Moment That Changed Everything
Watson’s win on Jeopardy! in 2011 was a big deal for AI. It showed how smart computers can be. Watson beat Brad Rutter and Ken Jennings, proving it can understand and process human language like a virtual assistant. This was thanks to deep learning and natural language skills.
The competition was tough. Watson played 100 games against past winners to get ready. In the end, Watson won with a score of $77,147. This was more than Rutter’s $21,600 and Jennings’ $24,000. Watson’s victory was a big step forward for AI, leading to more investment in the field.
Watson’s success on Jeopardy! was more than just a show. It showed its power to change industries like healthcare and finance. Watson can analyze lots of data and give accurate answers. It could become a key virtual assistant in many areas, getting better with deep learning.
Category | Watson’s Score | Rutter’s Score | Jennings’ Score |
---|---|---|---|
Final Score | $77,147 | $21,600 | $24,000 |
Understanding Watson’s Core Technologies
Exploring IBM’s Watson means diving into its core technologies. Watson uses natural language processing, machine learning, and data analytics. These tools help it handle and analyze huge data sets. This is great for cloud computing, where lots of data can be stored and processed easily.
Watson can understand and interpret human language. This makes it perfect for chatbots and virtual assistants. Its machine learning lets it get better with time, a key part of artificial intelligence. Watson can offer insights and help make decisions in many fields.
Watson’s main features are:
- Natural language processing capabilities
- Machine learning architecture
- Data analytics and processing power
These features help Watson analyze big data, spot patterns, and suggest actions. It’s a strong tool for businesses and organizations.
Knowing Watson’s core technologies opens up its full power. It can help improve customer service, optimize operations, or drive business insights. Watson’s mix of natural language processing, machine learning, and data analytics makes it a top choice.
Technology | Description |
---|---|
Natural Language Processing | Enables Watson to understand and interpret human language |
Machine Learning | Allows Watson to learn from data and improve its performance over time |
Data Analytics | Enables Watson to analyze large amounts of data and provide insights |
Early Commercial Applications and Expectations
Exploring ibm’s watson reveals its early uses and hopes. At first, Watson’s uses were few, facing challenges. The setup cost was high, around $3 million, and it took a few seconds to answer questions.
Despite these hurdles, Watson was expected to excel in oncology by 2013. This was two years after it started learning. The medical field was a key area, as medical info grows fast. Cognitive computing was seen as a game-changer for doctors.
Watson was expected to make a big difference in several areas:
- Medical diagnosis and research
- Financial analysis and planning
- Customer service and support
Looking at ibm’s watson, cognitive computing could change many industries. Watson’s ability to handle huge data sets and offer insights is exciting. It marks a big step in artificial intelligence.
Healthcare Ambitions: Watson’s First Major Industry Focus
IBM’s Watson made a big splash in the healthcare world early on. It teamed up with Memorial Sloan Kettering to tackle huge medical data sets. Watson used machine learning to get smarter and help doctors make better choices.
The aim was to make patients healthier and save money. Watson looked through lots of data to find patterns that could guide treatments. But, using Watson in real healthcare settings proved harder than expected. It found it tough to diagnose diseases, like cancer, which has many genetic factors.
Even with these hurdles, Watson’s impact on healthcare could be huge. Studies say AI could boost treatment success by 30 to 40 percent. It could also cut healthcare costs by up to 50 percent. As healthcare keeps changing, it will be exciting to see Watson and AI make a difference.
The Technical Limitations IBM’s Watson That Held Watson Back
IBM’s Watson had a promising start but faced big technical hurdles. Its natural language processing was advanced but not enough for complex tasks, like in healthcare.
Watson struggled with common sense and needed structured data. Users were unhappy, doubting Watson’s advice. Its cognitive computing was impressive but not ready for human-like decisions.
Some key issues Watson faced include:
- Lack of common sense and real-world experience
- Reliance on structured data
- Insufficient natural language processing capabilities
- Limitations in cognitive computing capabilities
These problems affected Watson’s performance, mainly in healthcare. Despite this, IBM kept working on Watson to improve its abilities.
Watson’s story shows its technical limits were a big problem. Yet, the lessons from Watson will help improve cognitive computing and natural language processing. This will lead to more advanced AI systems.
IBM’s Watson was once hailed as a groundbreaking achievement in artificial intelligence, especially after its dramatic victory on the quiz show Jeopardy! However, behind the impressive branding and marketing lay significant technical limitations that ultimately hindered its ability to fully realize its potential. One of the primary challenges was Watson’s reliance on structured data and predefined, narrow domains for training; this made it difficult for the system to adapt to the highly variable nature of human language and the broader spectrum of knowledge necessary for real-world applications. As a result, while IBM’s Watson excelled in certain contexts, its performance often faltered outside those boundaries.
Another obstacle was the complexity of natural language processing (NLP). Although Watson showcased remarkable capabilities in understanding and generating human-like text, its grasp of context and nuance was not as robust as one would expect from a system designed to interact with users. This limitation often led to misunderstandings or inaccuracies in its responses, causing frustration for users seeking more nuanced or contextually appropriate answers. As a result, many organizations were hesitant to adopt IBM’s Watson for critical tasks where precision was paramount, keeping it from becoming the ubiquitous force in AI that IBM envisioned.
Despite these setbacks, the story of IBM’s Watson serves as a valuable case study in the evolution of AI technology. The experiences and insights gained from working with Watson highlight the importance of addressing the challenges of cognitive computing and advancing natural language processing techniques. By learning from Watson’s limitations, researchers and developers can refine their approaches, fostering the development of more sophisticated AI systems that can understand and interact with the complexities of human language more effectively. These lessons are paving the way for future innovations that will ultimately bridge the gap between human intelligence and machine learning.
In light of these challenges, the journey of IBM’s Watson is not one of failure but rather a stepping stone towards a deeper understanding of what it takes to build intelligent systems. The lessons learned from its technical constraints will undeniably influence the next generation of AI, ensuring that future systems are better equipped to handle the intricacies of human communication and decision-making. This evolution will lead to advancements that can create AI platforms capable of serving industries more efficiently, driving us closer to a future where cognitive computing plays an integral role in our daily lives.
Competition Heats Up: How Other AI Platforms Gained Ground
The AI market has become more competitive. Cloud-based AI services and specialized AI solutions have emerged. This is due to the increasing need for artificial intelligence and deep learning.
The market is expected to grow fast, with a 30.1% annual growth rate from 2024 to 2030. The Artificial Neural Network Software Market is set to reach USD 20 Billion by 2030. This growth is fueled by big data and cloud computing, making it a great time for AI investments.
Platforms like Salesforce Einstein and Zendesk are making waves. Salesforce Einstein boosts conversion rates by personalizing customer interactions. Zendesk reduces service request handling time. These examples show AI’s power in improving business and customer experience.
Several factors are driving AI adoption:
- Availability of big data
- Cloud computing
- Strategic partnerships and investments in research and development
As the AI market evolves, staying updated is key. Knowing the competition and AI demand helps in making smart business decisions.
Watson’s Pivot to Enterprise Solutions
ibm’s watson evolved and shifted its strategy to focus on enterprise solutions. It aimed to offer business analytics and customer service, using machine learning to boost growth.
This change was due to the market’s evolution and the need for ibm’s watson to adapt. Watson’s advanced machine learning made it perfect for providing solutions to businesses. These solutions help companies make better decisions based on data.
- Business analytics: It finds insights and patterns in big data to guide business choices.
- Customer service: It uses machine learning for personalized and quick customer support.
Watson’s machine learning powers its enterprise solutions. These solutions help businesses grow and satisfy customers better. As AI in business grows, Watson’s move to enterprise solutions is timely and fitting.
The Marketing Challenge: Managing Public Perception
When thinking about cognitive computing and artificial intelligence in business, managing public perception is key. IBM’s Watson is a great example. It faced big marketing challenges in meeting public expectations. The company’s big marketing push raised high hopes about Watson’s abilities.
IBM has made over 4,000 marketing assets with its Creative Assistant. This shows AI’s power in making content. But, it also shows the need to manage public expectations well. The aim is to train 2 million people in AI by 2026. This will help solve the problems of big organizations.
Some reasons for unrealistic expectations include:
- Too much focus on AI’s possibilities
- Not understanding AI’s limits
- Not clearly sharing what the tech can really do
By facing these challenges and working on them, companies can improve how they’re seen. This way, their marketing can really show off the good things about cognitive computing and AI.
Marketing Challenge | Impact on Public Perception |
---|---|
Unrealistic Expectations | Less trust in the tech |
Lack of Understanding | Confusion about what the tech can do |
Insufficient Communication | Expectations don’t match reality |
Current State of Watson Technology
IBM’s Watson technology has seen big steps forward in machine learning and natural language processing. It can now handle huge amounts of data. This makes it very useful for many industries.
Watson can learn from data, making it a top choice for businesses using AI. It keeps getting better, with new features added often. For example, the Granite code models make Watson better at understanding natural language and SQL.
Recent Developments
IBM updated Watsonx at the THINK conference in May 2024. This update makes Watson even more powerful for businesses. Also, working with Red Hat makes it easier to use Watson in hybrid environments.
Active Implementation Areas
Watson is being used in many areas, including:
- Automation, which could lead to 1 billion applications by 2028
- AI tools like IBM Concert, coming out in June 2024
- Hybrid environments, where Watson makes AI easier to use
The economic benefits of AI, including Watson, could reach $4 trillion annually. Looking at Watson now, it’s clear machine learning and natural language are key to its growth and use.
Technology | Description |
---|---|
Granite code models | Enable Watson to outperform other open-source code models |
Watsonx platform | Updated to enhance Watson’s capabilities |
RHEL AI | Tool used to simplify AI deployment across hybrid environments |
Lessons for the AI Industry
When thinking about artificial intelligence, learning from pioneers like IBM’s Watson is key. Watson’s story teaches us about managing expectations and finding real uses for AI. It shows how important it is to make AI work in practical ways.
One big lesson is to understand how AI will affect industries and businesses. We must know its limits and challenges. This way, our AI projects will match our company’s goals.
For example, using artificial intelligence credentials can help meet the demand for AI skills. Also, AI tools like Watson can save a lot of time and money by finding expert knowledge faster.
Key takeaways for the AI industry are:
* Manage expectations and find real uses for AI
* Know the limits and challenges of AI
* Make sure AI projects fit with company goals
* Invest in AI skills and talent for the industry’s growth
Future Possibilities for Watson
Thinking about the future of ibm’s watson is exciting. Its machine learning skills make it useful in many fields, like healthcare and finance. A study on pmc.ncbi.nlm.nih.gov shows Watson could make medical decisions better and help patients more.
Watson could be used in many ways, such as:
- Creating personalized treatment plans in healthcare
- Improving financial analysis and forecasting in finance
- Optimizing supply chains in logistics
Watson’s power to handle lots of data and learn from it makes it a game-changer. As machine learning grows, Watson’s uses will too.
Here’s how Watson could help in healthcare:
Application | Description |
---|---|
Drug Discovery | Watson can sift through data to find new drugs and treatments. |
Personalized Medicine | Watson can help doctors tailor treatments to fit each patient’s needs. |
Conclusion: The Legacy of Watson and the Future of AI
IBM’s Watson AI system has made a big impact on artificial intelligence and cognitive computing. It has faced challenges, but Watson’s legacy is shaping the future of AI.
Watson’s win on “Jeopardy!” in 2011 was a big moment. It showed AI’s ability to solve complex tasks. This victory sparked a lot of innovation and investment in AI.
Watson’s work will keep influencing AI as it grows. It will help improve how humans and machines work together. Watson’s technology will also lead to better insights and predictions in many fields.
But, there are challenges ahead, like dealing with ethics and making sure AI is useful. Watson’s legacy shows the power of artificial intelligence. Looking forward, AI’s future is bright, and Watson’s journey will help guide us towards a smarter, more collaborative world.
0 Comment